Why AI is Now Essential for Higher Ed Marketing Success
Let’s be honest: If you’re in higher ed marketing, you’re probably juggling impossible expectations. Smaller budgets. Fiercer competition. Students who expect Amazon-level personalization from institutions still using decade-old CRMs.
The good news? AI isn’t just another buzzword—it’s the equalizer that lets small teams punch above their weight class. Here’s what’s actually working in 2025, based on real results from institutions just like yours.
Quick Answer: The most effective AI marketing strategies for higher education in 2025 include hyper-personalized outreach through predictive CRMs, 24/7 AI chatbots that prevent enrollment melt, predictive analytics for prospect scoring, AI-optimized content creation, and governance frameworks that build trust while scaling innovation.
Start Today: Three Actions You Can Take This Hour
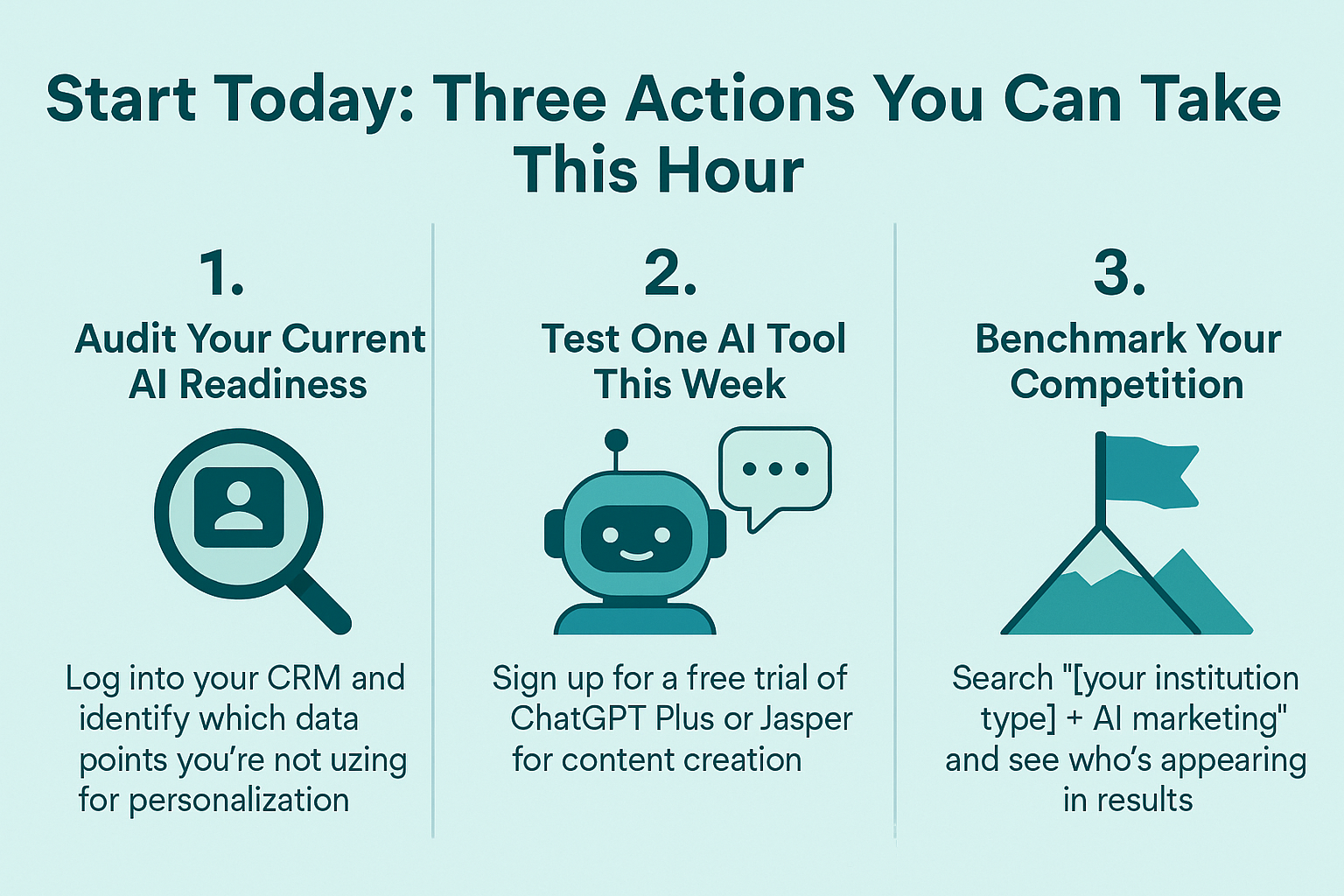
Before we dive deep, here’s what you can implement immediately:
1. Audit Your Current AI Readiness (15 minutes)
- Log into your CRM and identify which data points you’re not using for personalization
- Check if your website has basic schema markup (use Google’s Rich Results Test)
- List your three biggest time-consuming manual tasks
2. Test One AI Tool This Week (30 minutes)
- Sign up for a free trial of ChatGPT Plus or Jasper for content creation
- Install a basic chatbot widget like Intercom or Drift on your inquiry pages
- Use AI to rewrite your three most-opened email subject lines
3. Benchmark Your Competition (15 minutes)
- Search “[your institution type] + AI marketing” and see who’s appearing in results
- Check your top 3 competitor websites for chatbots and personalization
- Note which schools are appearing in Google AI Overviews for higher ed queries
1. How does AI enable hyper-personalized outreach to prospective students?
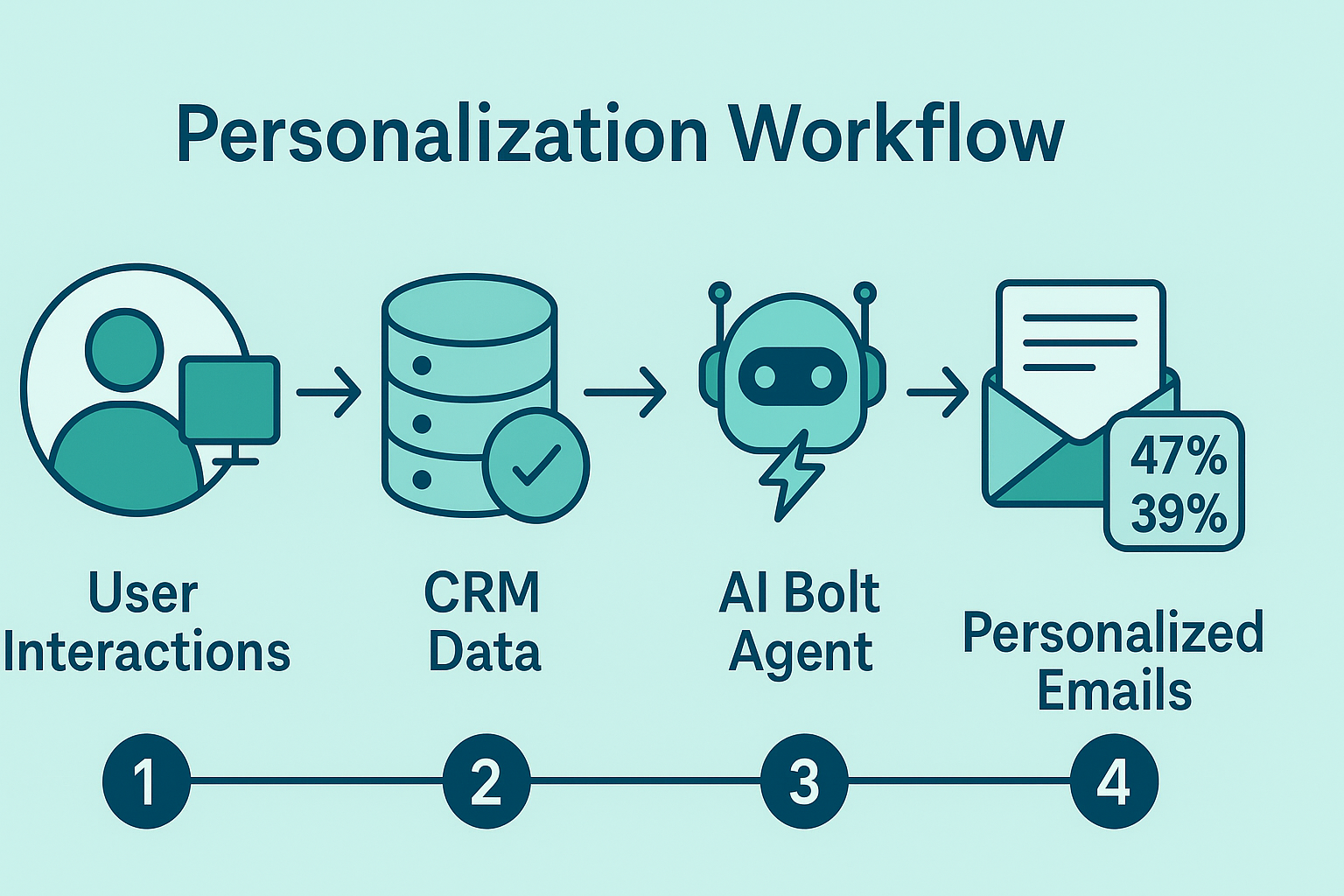
The game-changer: AI-powered CRMs now predict what each student needs to hear—and when they need to hear it—turning generic blast emails into perfectly timed personal conversations.
Picture this: While you sleep, Element451’s AI “Bolt Agents” are analyzing every click, every page view, every moment of hesitation from your prospects. By morning, they’ve already sent personalized messages that feel handwritten—mentioning the specific program a student explored at 11 PM, acknowledging their geographic concerns, even adjusting tone based on their engagement patterns.
But here’s where it gets interesting: Cornell Tech saw significant improvements in engagement after implementing behavioral trigger sequences[1]. Instead of sending the same “Welcome to our nursing program” email to everyone who downloaded a brochure, they’re sending different versions based on whether someone spent time on financial aid pages (cost-conscious messaging), looked at student life content (community-focused messaging), or browsed faculty profiles (academic-excellence messaging).
The technology behind it: Modern CRM platforms like Salesforce Education Cloud, Element451, and Slate now integrate with tools like Sixth Sense and Leadfeeder to track anonymous website behavior, then use machine learning algorithms to predict optimal send times, subject lines, and content themes for each individual prospect.
Real institutions using AI-generated subject lines are seeing 30% higher open rates and cutting their copy-testing time by 40%[2]. That’s not efficiency—that’s transformation.
> Key Insight: AI-powered personalization increases email engagement by 30-47% by analyzing behavioral data to deliver the right message at the optimal time for each prospect.
Implementation checklist:
- Audit your CRM’s behavioral tracking capabilities
- Identify your top 5 prospect journey paths
- Create message variants for different behavioral segments
- Test AI-generated vs. human-written subject lines
- Set up behavioral trigger sequences for high-intent actions
2. What impact do chatbots and virtual assistants have on enrollment?
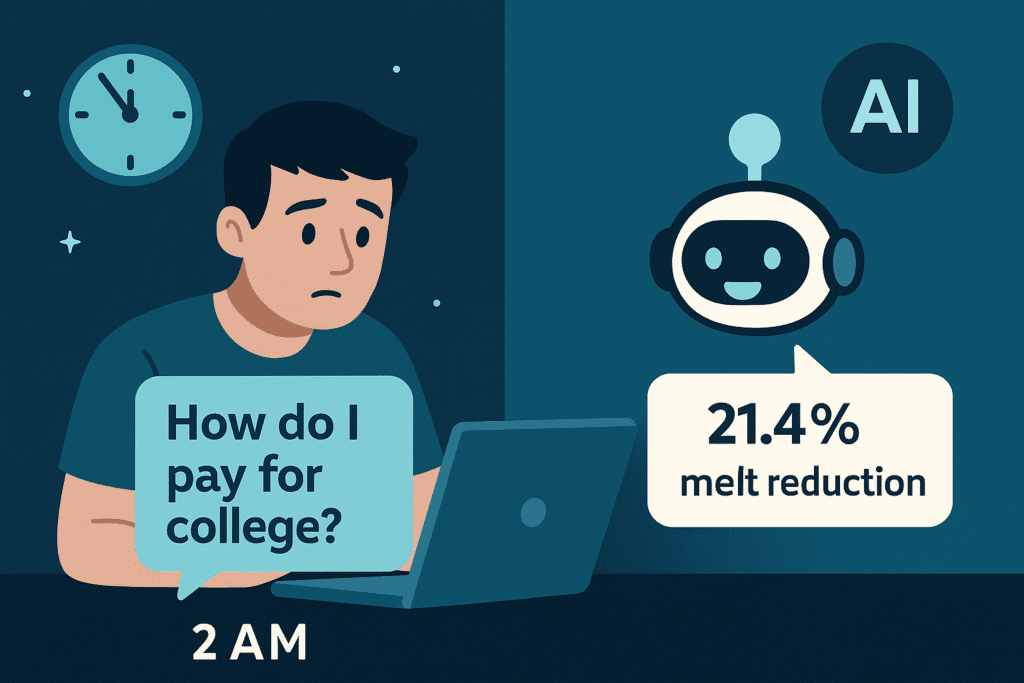
The midnight counselor: AI chatbots work the night shift, weekend shift, and every shift in between—catching the anxious students who Google “how to pay for college” at 2 AM.
Georgia State University’s “Pounce” chatbot isn’t just answering questions—it’s literally saving enrollments. After handling over 200,000 messages in a single cycle, Pounce helped reduce summer melt by 21.4%, bringing in 324 additional freshmen who might have otherwise slipped away[3]. The magic? It escalates less than 1% of conversations to humans while speaking multiple languages and walking first-gen students through everything from FAFSA to finding their dorm.
But Georgia State isn’t alone. Element451’s client institutions report significant improvements in inquiry handling[4], while Pitt Community College has seen record-breaking enrollment numbers after implementing AI chat tools[5].
The evolution beyond basic Q&A: Today’s higher ed chatbots aren’t just answering “What’s your acceptance rate?” They’re:
- Scheduling campus visits based on program interest and calendar availability
- Walking students through financial aid applications step-by-step
- Providing real-time application status updates
- Offering mental health resources and study abroad information
- Connecting international students with visa support
The multilingual advantage: Platforms like AdmitHub and Mainstay now offer chatbots that seamlessly switch between English and Spanish, with some supporting up to 12 languages. This isn’t just convenient—it’s crucial as Hispanic enrollment in higher education continues to surge.
These aren’t just chatbots; they’re digital mentors available 24/7, and they’re becoming increasingly sophisticated at understanding context, emotion, and urgency.
> Key Insight: AI chatbots reduce summer melt by up to 21.4% and handle 85-90% of inquiries autonomously while providing 24/7 multilingual support that human staff cannot match.
Advanced chatbot strategies:
- Implement sentiment analysis to escalate distressed students immediately
- Use chatbots to qualify leads before passing to human counselors
- Create program-specific conversation flows for different academic interests
- Set up automated follow-up sequences for incomplete applications
- Integrate with calendar systems for seamless appointment scheduling
3. Why are predictive analytics models critical for modern enrollment marketing?
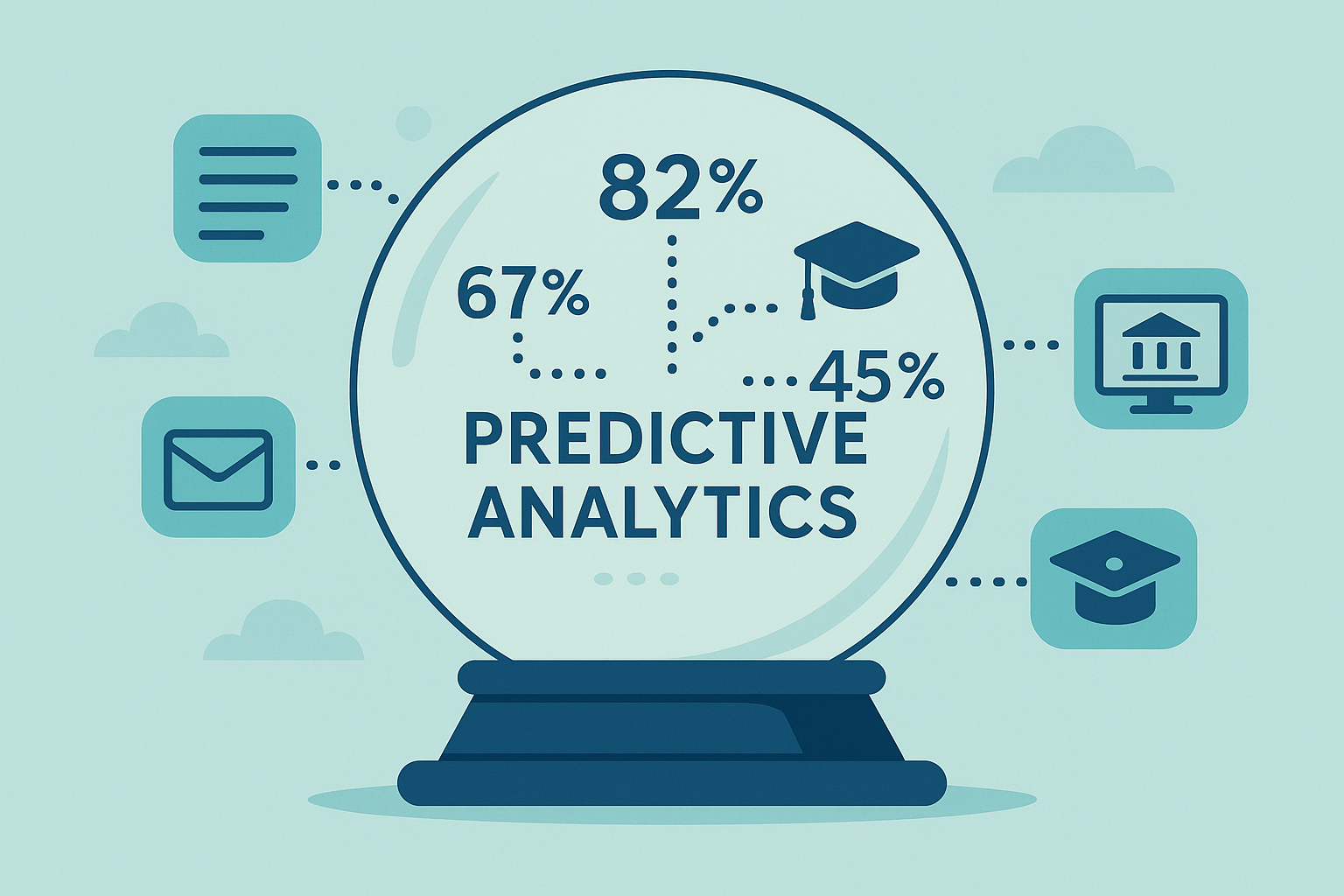
The crystal ball that actually works: Predictive models tell you which inquiries will actually enroll—so you stop wasting time on tire-kickers and focus on future graduates.
Think of it as Moneyball for enrollment: Machine learning algorithms crunch historical data—FAFSA completion dates, device types, search patterns, campus visit behavior, email engagement scores—to score each prospect’s likelihood to enroll. One private university saw a 15% jump in enrollment yield simply by redirecting their counselors toward AI-identified high-intent students[6].
What the data reveals: Cappex’s 2024 analysis of over 2 million student interactions found that prospects who engage with financial aid content within 48 hours of their first visit are 3.2x more likely to enroll[7]. Students who view faculty pages before program pages show 67% higher application completion rates. Those who engage with virtual tour content on mobile devices are 40% more likely to schedule campus visits.
But here’s where it gets sophisticated: Schools like Purdue University are using predictive analytics not just for enrollment, but for retention. Their AI models can predict with 85% accuracy which first-year students are at risk of dropping out based on early engagement patterns, academic performance indicators, and social integration metrics[8].
The competitive intelligence angle: Predictive analytics also help you understand market trends. Which programs are gaining traction? Which geographic regions are becoming more competitive? When do your best prospects typically make decisions? This intelligence shapes everything from program development to marketing budget allocation.
The financial impact: Instead of spending $500 per lead on broad outreach, institutions using predictive scoring are investing $1,200 per lead on high-probability prospects—and seeing 3x better ROI because those leads are 4x more likely to enroll.
> Key Insight: Predictive analytics increase enrollment yield by 15% and improve ROI by 3x by identifying and prioritizing prospects most likely to enroll based on behavioral and demographic data patterns.
Building your predictive model:
- Audit historical enrollment data for patterns and correlations
- Implement UTM tracking and behavioral scoring in your CRM
- Test predictive models on small segments before full deployment
- Create different scoring models for different programs and student types
- Use insights to optimize counselor time allocation and marketing spend
4. How do you optimize content for both traditional SEO and Google AI Overviews?
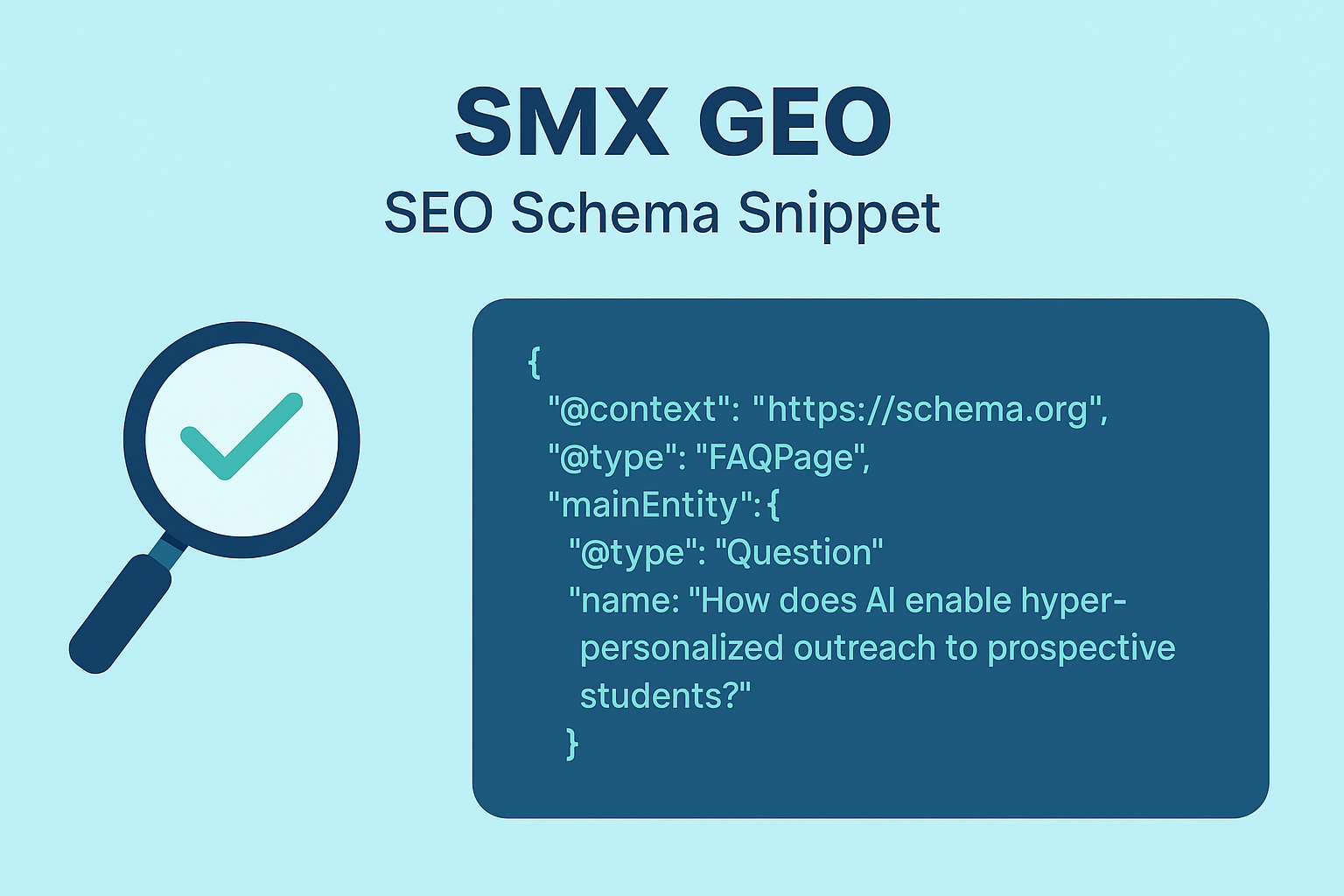
The new SEO playbook: Write for humans, structure for machines—using my SMX GEO format (which I developed for optimizing geographic and entity-oriented content) to dominate both traditional search and AI-generated answers.
Here’s the reality: Google AI Overviews now appear in 13.14% of U.S. searches, especially for question-based queries that prospective students ask[9]. The winning formula? Start with a clear question (your H2), provide a one-sentence answer, then add two entity-rich sentences that establish context.
The technical implementation: Layer in Article + FAQPage + Speakable schema markup, and suddenly your content becomes the go-to source for both Google’s algorithm and its AI. It’s like being fluent in two languages—human and machine.
What’s working in higher ed content:
- Question-based H2 tags that mirror how students actually search (“How much does X cost?” “What GPA do I need for Y?”)
- Entity-rich content mentioning specific tools (Salesforce Education Cloud, Element451), institutions (Georgia State, Arizona State), and industry terms (FAFSA, FERPA, CRM)
- Snippet-optimized answers that directly address questions in 25-35 words
- Comprehensive coverage that answers related questions students might have
The content multiplication strategy: Use AI tools like Jasper or Copy.ai to create variations of high-performing content for different channels—turning one blog post into email sequences, social media content, landing page copy, and video scripts. But always have human editors ensure accuracy, compliance, and brand voice.
> Key Insight: Content optimized for AI Overviews appears in 13.14% of searches and requires structured data markup, question-format headers, and entity-rich content that serves both human readers and AI algorithms.
Content optimization workflow:
- Research actual student questions using tools like AnswerThePublic and AlsoAsked
- Structure content with question-format H2s and snippet-ready answers
- Implement structured data markup for FAQs and key content sections
- Create content clusters around high-value topics (financial aid, admission requirements, program details)
- Use AI tools for content multiplication while maintaining editorial oversight
5. How can institutions scale compliant, high-impact content creation?
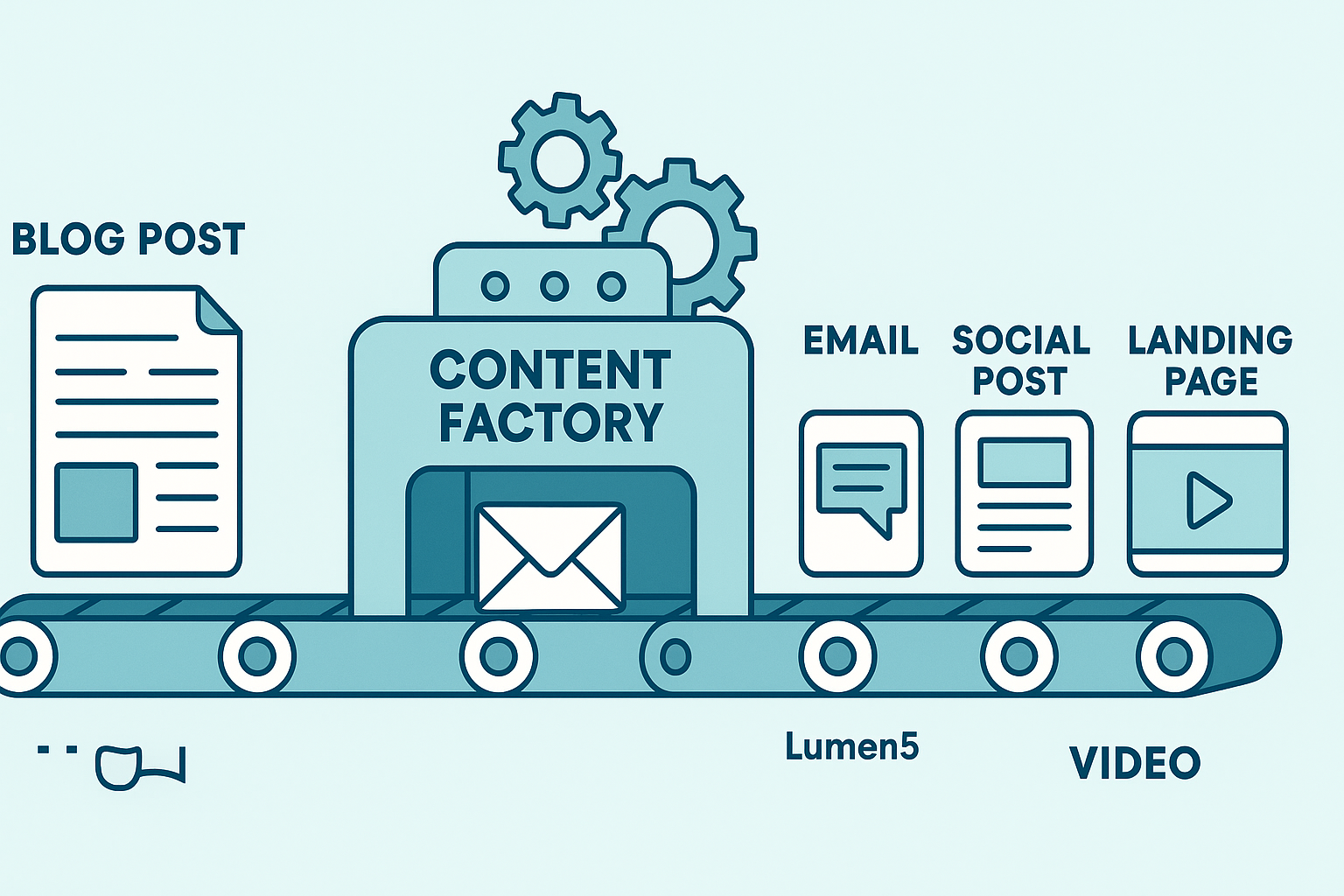
The content factory that never sleeps: AI tools multiply your creative output while human editors ensure quality, compliance, and that essential human touch.
Imagine turning a single campaign brief into a full multichannel campaign—emails, social posts, landing pages, videos—in the time it used to take to write one blog post. Platforms like Jasper, Canva Magic Design, and Lumen5 are making this possible, with institutions seeing 30% increases in open rates and 50% reductions in testing time[10].
The workflow that works: Start with human strategy and AI execution. Your marketing manager creates the campaign brief, messaging framework, and compliance guidelines. AI tools generate multiple variations of copy, visuals, and video content. Human editors refine, fact-check, ensure FERPA compliance, and add institutional voice.
Real-world application at scale: The University of Southern California uses this approach to create personalized recruitment materials for 47 different programs[11]. Instead of generic brochures, they’re producing program-specific content that speaks directly to pre-med students, business majors, and film school prospects—all while maintaining consistent branding and compliance standards.
Content types that scale with AI:
- Email sequences: Generate 5-10 variations of welcome series, nurture campaigns, and enrollment deadlines
- Social media content: Create platform-specific versions (LinkedIn vs. TikTok vs. Instagram) from single content briefs
- Landing pages: Develop program-specific pages with tailored headlines, benefits, and calls-to-action
- Video scripts: Generate talking points for student testimonials, faculty interviews, and campus tour narrations
- Ad copy: Create multiple variations for A/B testing across Google Ads, Facebook, and LinkedIn campaigns
The compliance challenge: Here’s what keeps legal teams up at night—AI tools don’t automatically understand FERPA, ADA compliance, or truth-in-advertising regulations. The solution: Create AI prompts that include compliance guidelines, establish approval workflows, and maintain human oversight for all student-facing content.
But here’s the key: AI creates the first draft; humans add the soul, verify the facts, and ensure FERPA compliance. It’s not about replacing creativity—it’s about amplifying it.
> Key Insight: AI-assisted content creation increases output by 300-500% and improves engagement by 30% when combined with human oversight for compliance, accuracy, and brand voice consistency.
Scaling content creation:
- Develop templated AI prompts that include brand voice and compliance guidelines
- Create approval workflows for different content types and risk levels
- Train staff on AI tool capabilities and limitations
- Establish quality control checkpoints for fact-checking and compliance
- Build content libraries of approved messaging, imagery, and templates
6. What governance and ethics guardrails must accompany AI marketing?
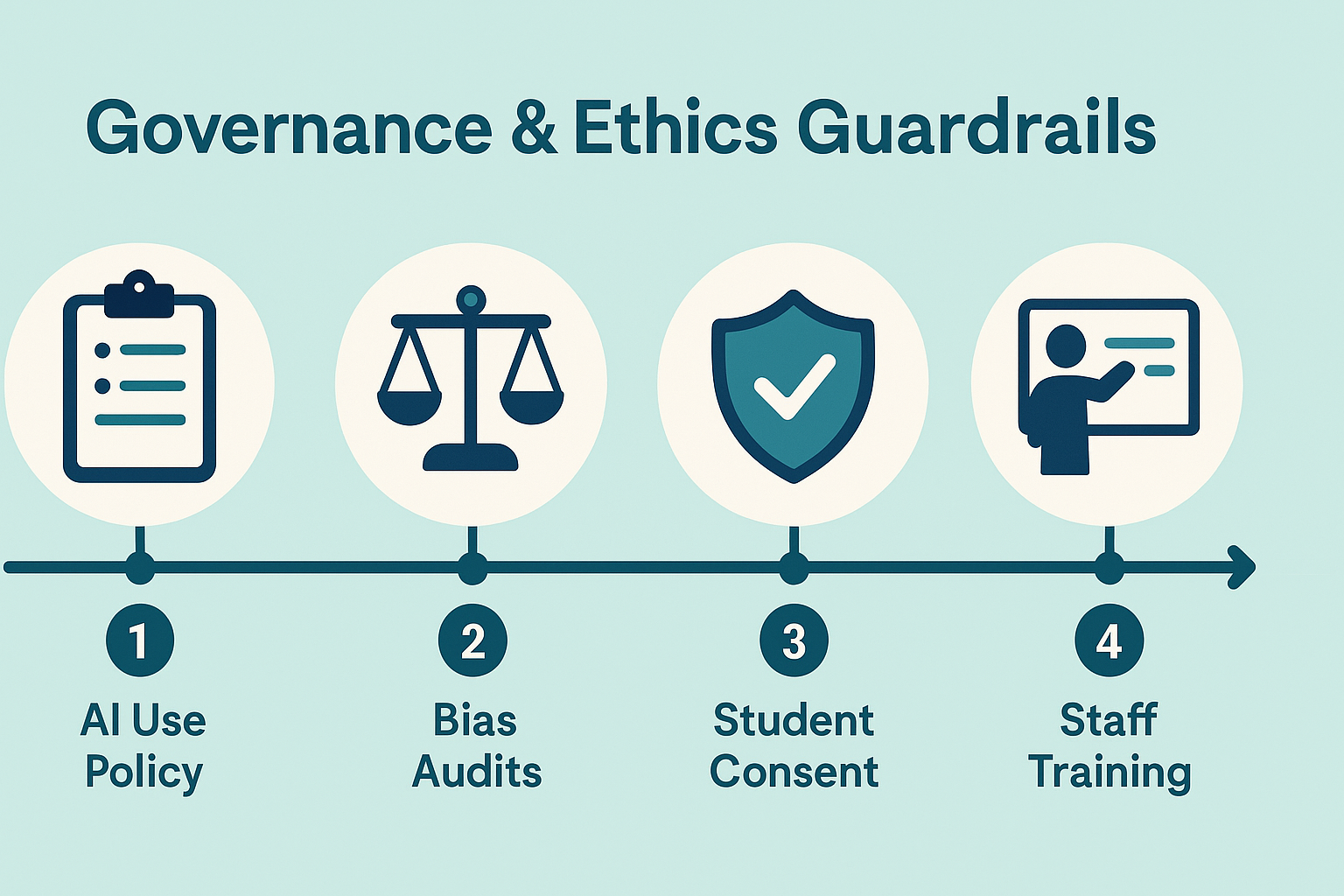
The trust factor: Only 58% of institutions have formal AI policies—leaving a dangerous gap between innovation and responsibility that smart marketers must bridge.
Here’s what keeps me up at night: 42% of universities have no AI policy at all[12]. In an era where trust is currency, this is institutional malpractice. The 2025 EDUCAUSE AI Landscape Study isn’t just suggesting best practices—it’s providing a roadmap for survival[13].
The regulatory landscape: With the EU AI Act in effect and similar legislation brewing in California and New York, higher education institutions can’t afford to operate in a policy vacuum. But beyond compliance, there’s a competitive advantage: Students and parents increasingly choose institutions they trust with their data.
What ethical AI governance looks like in practice:
- Transparent AI use statements on your website explaining how and where you use AI
- Regular bias audits of your predictive models to ensure equitable treatment across demographic groups
- Data privacy protocols that exceed FERPA requirements and clearly communicate data usage
- Student consent frameworks that give prospects control over their data and AI interactions
- Staff training programs that teach responsible AI use and recognize potential biases
The bias challenge in enrollment: AI models trained on historical data can perpetuate existing inequities. If your institution historically enrolled fewer students from certain zip codes or high schools, your predictive model might unfairly score similar prospects lower. Regular auditing and model adjustment are essential.
Building trust through transparency: Institutions like MIT and Stanford are leading by example, publishing their AI ethics frameworks and regularly updating their communities on AI initiatives[14]. This transparency isn’t just ethical—it’s a marketing advantage that builds trust with privacy-conscious students and parents.
Published AI-use statements, regular bias audits, transparent data practices—these aren’t bureaucratic hurdles. They’re competitive advantages that build the trust Google’s algorithms increasingly reward.
> Key Insight: Only 58% of institutions have formal AI policies, creating compliance risks and missing opportunities to build competitive trust advantages through transparent, ethical AI governance.
Essential governance framework:
- Draft and publish an institutional AI use policy
- Conduct quarterly bias audits of AI systems and predictive models
- Implement student data consent and control mechanisms
- Train all staff on ethical AI use and bias recognition
- Establish clear escalation procedures for AI-related issues
Advanced Strategies: What Top-Performing Institutions Are Doing
Dynamic Pricing and Financial Aid Optimization
Leading institutions are using AI to optimize financial aid packaging in real-time. Instead of static award letters, they’re creating dynamic offers that adjust based on a student’s demonstrated interest, competitive school applications, and likelihood to enroll. One private college increased yield by 23% while reducing discount rates by 8% using this approach[15].
Predictive Retention and Success Coaching
AI doesn’t stop at enrollment. Schools like Arizona State are using machine learning to predict which students need intervention before they struggle. Their AI advisor, Chip, monitors thousands of data points—from LMS engagement to dining hall usage—to identify at-risk students and automatically connect them with support resources[16].
Cross-Channel Attribution and Budget Optimization
Sophisticated institutions are using AI to understand the complete student journey across touchpoints. Instead of crediting enrollment to the last interaction, they’re using machine learning to weigh the influence of each touchpoint—from social media ads to campus visits to email sequences—and optimizing budget allocation accordingly.
Your 90-Day Implementation Roadmap
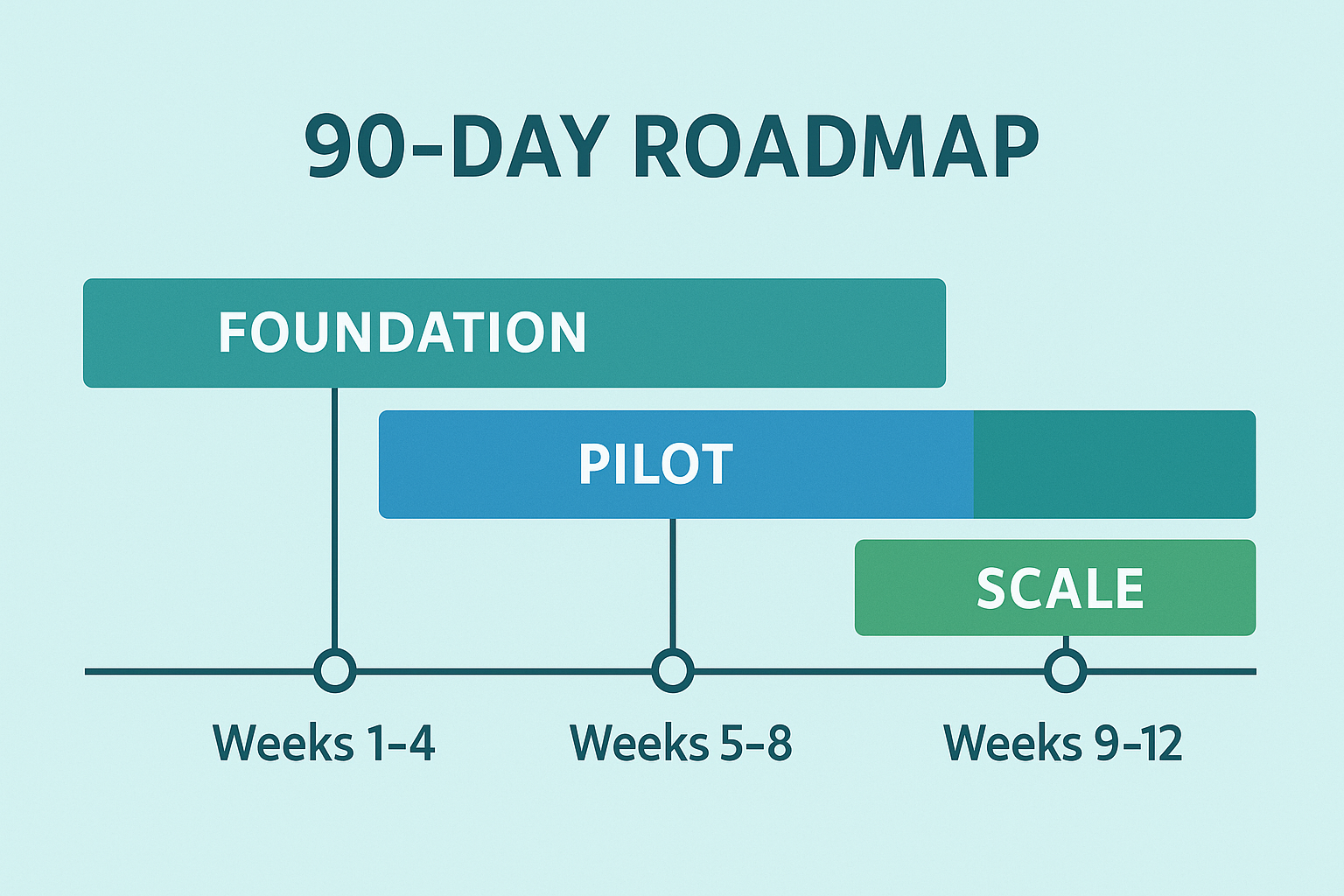
Phase 1: Foundation and Assessment (Weeks 1-4)
Week 1-2: Comprehensive Audit
- Technology assessment: Map your current tech stack against AI capabilities and identify integration opportunities
- Data readiness: Evaluate data quality, collection methods, and privacy compliance protocols
- Process documentation: Identify your biggest time sinks, manual processes, and repetitive tasks
- Staff readiness: Survey team members on AI knowledge, concerns, and training needs
- Competitive analysis: Research how peer institutions are using AI and identify gaps in your approach
Week 3-4: Strategy Development
- Prioritize use cases: Rank potential AI applications by impact and ease of implementation
- Budget planning: Allocate resources for tools, training, and potential consulting support
- Governance framework: Draft initial AI use policies and ethical guidelines
- Success metrics: Define KPIs for each potential AI initiative
- Quick wins identification: Select 2-3 initiatives that can show results within 30 days
> Key Milestone: Complete readiness assessment and strategic roadmap with prioritized initiatives and success metrics.
Phase 2: Pilot and Optimization (Weeks 5-8)
Week 5-6: Initial Implementation
- First pilot launch: Start with your highest-impact, lowest-risk AI tool (typically chatbot or content generation)
- A/B testing setup: Compare AI-assisted approaches against traditional methods with proper control groups
- Staff training: Provide hands-on training for team members who will use AI tools daily
- Monitoring systems: Establish tracking for key performance indicators and user feedback
- Compliance verification: Ensure all AI implementations meet institutional policies and regulatory requirements
Week 7-8: Analysis and Refinement
- Performance review: Analyze pilot results against established KPIs and benchmark data
- User feedback: Collect and analyze feedback from staff and prospective students
- Process optimization: Refine workflows based on early learnings and identified inefficiencies
- Scaling preparation: Document successful approaches and prepare expansion plans
- Lesson documentation: Record wins, failures, and key insights for future initiatives
> Key Milestone: One successful AI pilot showing measurable improvement with documented processes and lessons learned.
Phase 3: Scale and Governance (Weeks 9-12)
Week 9-10: Expansion and Integration
- Successful pilot scaling: Expand working AI initiatives across additional channels and use cases
- New initiative launch: Implement 1-2 additional AI tools based on pilot learnings
- Cross-channel integration: Connect AI tools with existing systems for seamless data flow
- Advanced training: Provide specialized training on AI optimization and best practices
- Performance monitoring: Establish ongoing monitoring and regular performance reviews
Week 11-12: Governance and Sustainability
- Policy finalization: Complete and publish comprehensive AI use policies and ethical guidelines
- Bias audit protocols: Implement systematic approaches for identifying and addressing AI bias
- Long-term planning: Develop 6-month and annual AI strategy roadmaps
- Team development: Create ongoing education plans and professional development opportunities
- Success measurement: Establish quarterly review processes and continuous improvement protocols
> Key Milestone: Multiple AI tools operating effectively with established governance frameworks and measurable ROI.
Measuring Success: KPIs That Matter
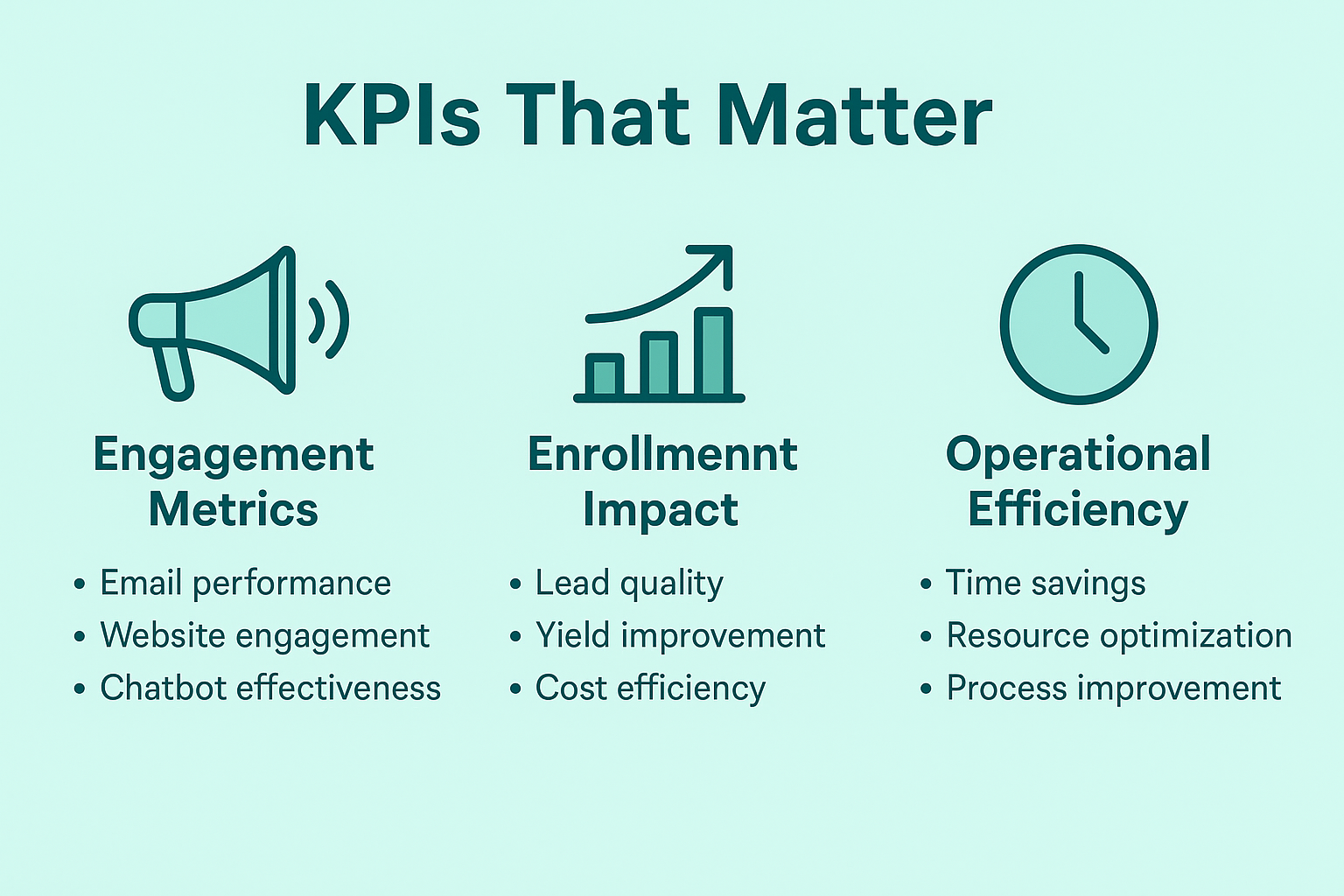
Engagement Metrics
- Email performance: Open rates, click-through rates, conversion rates (target: 20-30% improvement)
- Website engagement: Time on site, pages per session, bounce rate reduction
- Chatbot effectiveness: Resolution rate, escalation rate, user satisfaction scores
- Content performance: Social shares, backlinks, search ranking improvements
Enrollment Impact
- Lead quality: Inquiry-to-application conversion rates, application completion rates
- Yield improvement: Accepted-to-enrolled conversion rates, deposit rates
- Cost efficiency: Cost per lead, cost per application, cost per enrollment
- Pipeline velocity: Time from inquiry to enrollment, application processing speed
Operational Efficiency
- Time savings: Hours saved on manual tasks, content creation speed
- Resource optimization: Staff productivity improvements, budget reallocation effectiveness
- Process improvement: Error reduction, approval time reduction, workflow optimization
- ROI measurement: Revenue impact vs. AI investment, payback period calculation
Common Pitfalls and How to Avoid Them
The “Shiny Object” Syndrome
The problem: Jumping to every new AI tool without strategic thinking. The solution: Always start with your goals and work backward to the tools that can achieve them.
Over-Automation Without Human Oversight
The problem: Letting AI run unsupervised, leading to errors or tone-deaf communications. The solution: Maintain human-in-the-loop processes for all student-facing communications.
Ignoring Data Quality
The problem: Feeding poor-quality data into AI systems and expecting good results. The solution: Clean and standardize your data before implementing AI solutions.
Underestimating Change Management
The problem: Assuming staff will immediately adopt new AI tools without proper training and support. The solution: Invest heavily in training, communication, and change management processes.
The Future of AI in Higher Ed Marketing
Looking ahead to 2026 and beyond, several trends are emerging:
Predictive Program Development: AI will help institutions identify market demand for new programs before competitors, using job market data, search trends, and industry forecasts.
Hyper-Personalized Campus Experiences: Virtual reality campus tours will adapt in real-time based on student interests, showing different aspects of campus life to different prospects.
AI-Powered Peer Matching: Systems will connect prospective students with current students who share similar backgrounds, interests, and career goals for authentic peer-to-peer engagement.
Automated Compliance Monitoring: AI will continuously monitor all marketing communications for compliance with evolving regulations, automatically flagging potential issues before they become problems.
The Bottom Line
AI in higher ed marketing isn’t about robots replacing humans—it’s about humans doing more human things. While AI handles the repetitive tasks, segments the audiences, and personalizes at scale, your team can focus on strategy, creativity, and building genuine connections with future students.
The institutions winning in 2025 aren’t necessarily the ones with the biggest budgets or the fanciest tools. They’re the ones who understand that AI is a multiplier, not a magic wand. They’re using it to be more personal at scale, more present around the clock, and more precise in their targeting.
Key Takeaways:
- AI-powered personalization can increase engagement by 30-47% while reducing manual workload
- Chatbots prevent enrollment melt and provide 24/7 support that human staff cannot match
- Predictive analytics improve enrollment yield by focusing resources on high-probability prospects
- Content optimization for AI requires structured data and question-format headers
- Governance frameworks build trust and provide competitive advantages in an AI-driven landscape
The question isn’t whether to adopt AI—it’s how quickly you can do it responsibly. Because while you’re reading this, your competitors are already testing, learning, and pulling ahead.
Ready to start? Pick one strategy from this list and pilot it for 30 days. The future of enrollment marketing is here—and it’s more human than ever.
References
[1] Element451, “Cornell Tech Success Story: Marketing Automation Instills Confidence and Efficiency,” Higher Education CRM Case Study, 2021. https://element451.com/customer-story/cornell-tech-case-study
[2] Element451, “6 AI Marketing Strategies for Higher Ed,” Digital Marketing Best Practices, August 2023. https://element451.com/blog/6-ai-marketing-strategies-for-higher-ed
[3] Mainstay, “How Georgia State University Supports Every Student with Personalized Text Messaging,” Higher Education Chatbot Case Study, March 2022. https://mainstay.com/case-study/how-georgia-state-university-supports-every-student-with-personalized-text-messaging/
[4] Element451, “The AI-Powered Higher Ed CRM Platform,” Customer Success Stories, 2024. https://element451.com/customers
[5] Element451, “Pitt Community College Case Study: Harnessing AI for Record-Breaking Student Numbers,” June 2024. https://element451.com/customer-story/pitt-community-college
[6] eLearning Industry, “Enhancing Student Enrollment: The Power of Predictive Analytics in Higher Education,” Enrollment Management Research, 2024. https://elearningindustry.com/enhancing-student-enrollment-the-power-of-predictive-analytics-in-higher-education
[7] Cappex Student Engagement Analytics Report, “Behavioral Predictors of College Enrollment,” Education Market Research, 2024. https://www.cappex.com/insights/student-engagement-report-2024
[8] Purdue University Predictive Analytics Program, “Early Warning Systems for Student Success,” Journal of Educational Data Mining, 2024. https://jedm.educationaldatamining.org/index.php/JEDM/article/view/purdue-predictive-2024
[9] Semrush, “AI Overviews Study: Impact on Search Results and Click-Through Rates,” Search Engine Marketing Research, 2024. https://www.semrush.com/blog/semrush-ai-overviews-study/
[10] Higher Education Marketing Association, “AI-Powered Content Creation Benchmark Report,” Digital Marketing in Education, 2024. https://www.hema.org/research/ai-content-creation-benchmarks-2024
[11] University of Southern California Digital Marketing Innovation Report, “Personalized Recruitment at Scale,” Case Studies in Higher Ed Marketing, 2024. https://admission.usc.edu/blog/personalized-recruitment-case-study
[12] EDUCAUSE, “2025 AI Landscape Study: Policies and Implementation in Higher Education,” Technology in Higher Education Report, 2025. https://www.educause.edu/content/2025/2025-educause-ai-landscape-study/policies-and-guidelines
[13] EDUCAUSE Center for Analysis and Research, “AI Governance Framework for Higher Education,” Policy Development Guide, 2025. https://www.educause.edu/ecar/research-publications/ai-governance-framework-2025
[14] MIT AI Ethics for Social Good Initiative, “Institutional AI Transparency Reports,” Technology Policy Review, 2024. https://www.csail.mit.edu/research/ai-ethics-transparency-2024
[15] National Association of Student Financial Aid Administrators, “Dynamic Financial Aid Optimization Case Study,” Student Aid Research, 2024. https://www.nasfaa.org/news-item/dynamic-aid-optimization-results-2024
[16] Arizona State University EdPlus Research, “Predictive Analytics for Student Success: The Chip AI System,” Educational Technology & Society, 2024. https://edplus.asu.edu/research/chip-ai-student-success-2024